Communication between countries was once reliant on ships, pen and paper, and subject to unfortunate weather conditions. Then came the subsea cable.
After some delays, the first trans-Atlantic subsea cable became operational in 1859. And though its tenure was brief, its impact has survived to today.
The more than 450 subsea cables in service today span more than 1.3 million kilometers and enable the transmission of data within seconds. However, these mighty cables would be rendered obsolete if not for the estimated 1,400 cable landing stations (CLS) connecting them to dry land.
A CLS is a facility, usually located along a coastline or shoreline, responsible for taking data from a subsea cable and connecting it to terrestrial infrastructure.
It may be the wires that run across the sea, but arguably, it is the cable landing stations doing all the heavy lifting.
What is a cable landing station facility?
The CLS carries traffic from subsea cables to infrastructure, such as satellite links, fiber optic cables, and microwave towers. From there, the data makes its way to customers and/or data centers where it is stored, processed, and distributed.
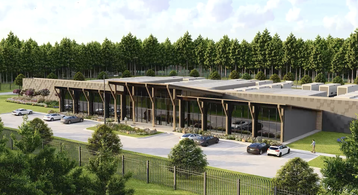
Quinalt Indian Nation CLS in Washington, US– Quinault Indian Nation | Toptana Technologies
Gil Santaliz, CEO of CLS operator NJFX, says that the stations are “where cables physically come out of the ocean,” as well as receiving power to transport data across the sea, with the data handed off to terrestrial networks within that country.
The amount of data being transmitted across those fibers can be as much as 40TB per second, and Santaliz says financially and logistically data cannot be transmitted from terrestrial cables to subsea ones without meeting at the CLS first.
The CLS provides the data with the shortest and most efficient route in and out of the country. Brian Lavallée, senior director at networking business Ciena, says: “Without submarine cables, and the data centers they interconnect, there would be no Internet today, as continents would be isolated islands of terrestrial connectivity.”
The facilities are also responsible for monitoring the cable status, ensuring there are no outages, and operating at peak performance.
Architecturally speaking, Thomas Fabre, senior director of network investment at Exa Infrastructure, says the landing stations we see today are not too dissimilar from small data center buildings located on the shoreline.
That is not to say a CLS is always a conventional permanent building. Modular data center provider DXN has been known to deploy modular cable landing stations in shipping container-type pods in locations across Australia and the Micronesian islands.
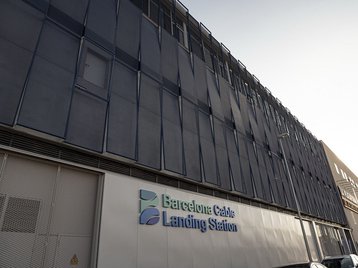
Barcelona cable landing station– Barcelona Cable Landing Station | AFR-IX Telecom
NJFX, in comparison, has an entire cable landing station campus, explains Santaliz. The company’s current CLS campus has seven doors and layers of security before reaching the actual facility. The building is “hidden in plain sight,” manned 24/7, and has more than 50 cameras across the site.
What happens inside a CLS?
When the cable leaves the ocean it enters beach manhole, which Exa’s Fabre describes as a “very large chamber, no more than 2x2x2 meters, where the cable is secured onto land.”
The cable then “continues to what we call the cable landing station, through a duct, which in the industry we call a fronthaul system,” he says.
Whilst Fabre says the fronthaul system can be located up to 20km away from the beach manhole, operators typically want the system as close to the manhole as possible to reduce potential for breakages or other errors.
The fronthaul system separates the cable to retrieve the copper core and the fiber. The copper core is channeled to the dry plant inside the CLS. Here, it is fed through the power-feeding equipment (PFE).
The PFE does what it says on the tin. Santaliz says the copper core will “hit that PFE and that copper core is going to be energized. That’s how you make sure the signal gets regenerated every 50km or so to make sure it travels across the ocean.”
Repeaters, explains Fabre, are laid “every 60 to 80 km along the subsea cable” and “amplify the optical signals so that it can reach from one shore to another.”
The CLS also has repeaters, found inside the wet plant, to allow the fiber pairs to be rerouted to other destinations and other landing points.
Where there is a fronthaul system, there is also a backhaul system. The backhaul system offloads the data to terrestrial networks connected to the CLS, ending up eventually at a data center where the data is stored, processed, and redistributed.
What is an open cable landing station?
To understand what an open CLS is, we need to go back in time, says Fabre.
He says: “Historically, the cables were greeted by a consortium of telecommunications companies, and back in those days, there were only incumbent telcos, like BT, Orange, Telefonica, and Telecom Italia.
“They would team up together and share the cost of the investment where the cable landed in a particular country. For instance, in the UK, it would have been BT who operated the CLS close to the shore and provided a backhaul to send the traffic from the CLS to the largest city where there is a data center.”
This was what was known as a closed system. In contrast, an open cable landing station is when a cable lands at the CLS and has multiple routes out of the station via various network operators and different terrestrial fiber options. Fabre says an open cable landing station can be thought of “like a carrier-neutral data center.”
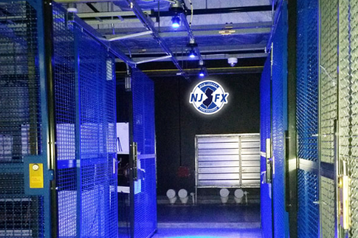
Inside NJFX’s cable landing station– New Jersey Fiber Exchange
Lavallée adds that open cable systems “allow cable operators to choose the wet plant – the network equipment on the seabed – from one vendor and the submarine line terminal equipment from another.” This creates a more competitive environment to accelerate technological innovation, he explains.
Santaliz added that these buildings were typically only made for one cable system. Modern CLS can host multiple cables in one facility.
NJFX’s business model means that the owner of the CLS does not necessarily take an ownership stake in the cable; the CLS owner remains neutral.
“In our case, the cable can leave 30, 40, or even 50 ways out of the cable landing stations. And our numbers are about 35 network operators and 20 plus terrestrial fiber cables coming in the other side of that cable station,” she says.
Santaliz argues this provides the cable system with diversity and resilience to traverse entire countries.
Where are cable landing stations located?
Cable landing stations cannot be just plonked anywhere. They must be located on stretches of coastline where cables can land safely without getting damaged.
Santaliz says cables can get damaged when rocks fall from underwater mountain ranges. “You have seismic activity, and then cables end up getting cut and rubbing on the edge of these mountain ranges,” he explains. “They get frayed, and they get compromised.”
Operators, therefore, have to be selective about where to construct their landing stations. Typically, they are built along coastlines with gently sloping and sandy seabeds, so they can be buried.
Marine traffic is also a risk to cables, so criteria are in place to ensure the cables will not be disturbed by ships or trawler operations.
Santaliz explains there is also a risk in choosing a new location to build a cable landing station.
“You might not be lucky,” he says. “And you might have cables that are being cut every year.”
Somewhere like New Jersey, in comparison, is a stable and proven location to land subsea cables, explains Santaliz.
“New Jersey has been hosting cables since the 1800s. In the US, it’s a proven territory where cables can cross the Atlantic Ocean,” he says.
Santaliz adds that Myrtle Beach in South Carolina looks like a good spot to build a cable landing station, but “the jury is still out” because the city’s existing CLS is only a year old.
US data center firm DC Blox developed the first CLS on Myrtle Beach, and has plans for a second in the pipeline. Google and Meta have both reserved slots at the facility.
How close does a CLS have to be to a data center?
Cable landing stations have been typically located in close proximity to data centers, allowing for the easy transmission of data.
Lavallée says that landing stations have been historically close to network hubs to facilitate efficient connectivity to population centers, but now the focus is on being close to hyperscale data centers.
Fabre notes that in the data center hub of Marseille, there is “a technical solution” to land your cable directly at a data center. However, the disadvantage to this solution is creating a “monopoly” on that cable, whereby it becomes a closed system again, only having access to one operator.
NJFX says its campus avoids such disadvantages by operating what it calls “a cable landing station colocation campus.” It incorporates a CLS and a data center in one site, and the backhaul system is therefore not required to trolley the data to the nearest data center. Instead, the processing and distribution all take place directly at the CLS.
But what happens if there is no data center near the CLS? In this instance, an open CLS is all the more important, Fabre says.
Exa Infrastructure is currently expanding its CLS in Genoa; a city with very little data center presence.
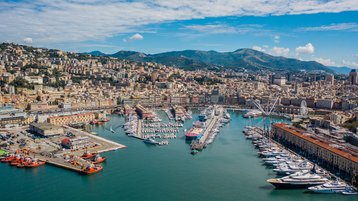
Genoa– Getty Images
“Most of the traffic in Genoa comes from Milan, which is some 150km away,” Fabre says. Adding more routes out of the Genoa CLS ensures Internet resiliency for the city. If one cable were to go down between Genoa-Milan, there would be other routes for securing connectivity.
Another reason for expanding the Genoa CLS lies in the fact that cables now require bigger backhaul systems to offload larger amounts of data. Fabre adds that previous cables only had eight fiber pairs. Nowadays, we are seeing 16 to 24 fiber pairs per cable.
What next for the CLS?
The first dedicated cable landing station was built in 1850 in Valentia Island, Ireland. Today, Egypt, Marseille, Tokyo, and Singapore are some of the biggest hubs hosting the most cables.
Marseille was traditionally thought of as the gateway to Europe and was a preferred location for cables because it is also a hub for data centers. In Asia, Singapore has 29 cables planned and operational landing on the small island. The country announced plans to double its submarine capacity in June last year. Egypt is also a key location for submarine cables. Around 17 percent of the world’s Internet traffic passes through the country.
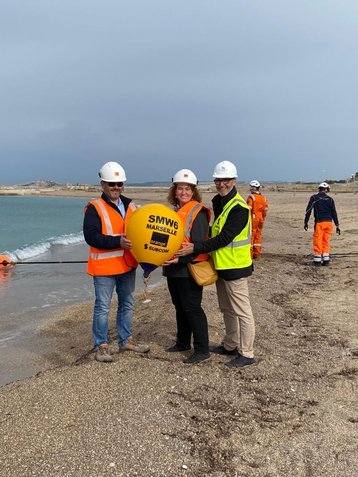
The SeaMeWe-6 cable landing in Marseille– Rohitash Bhaskar on LinkedIn
For Santaliz, AI is going to change the CLS game. The NJFX campus is designed as a data center that hosts cables, and soon other operators may catch on, meaning that the campus has the capability to host AI applications at the CLS.
He says: “The next logical step in the development of AI will be Edge AI. And it just so happens that NJFX was designed as a data center, so we’ll have 5MW of IT capacity to support the AI [inference] workloads of one or two customers.”
Fabre says we are seeing more and more fiber pairs per submarine cable, adding that such demand requires increasingly large backhaul systems. He also says it is becoming rare to see the launch of cables without a hyperscaler on board, particularly in Europe. He said part of this is because operators still want to be as close to a data center as possible, and having a hyperscaler on board the project means you are guaranteed to have somewhere to backhaul the traffic to.
What is clear is that for as long as subsea cables traverse the seas, cable landing stations are not going anywhere.